The Ultimate Guide to Data Annotation Tools and Platforms
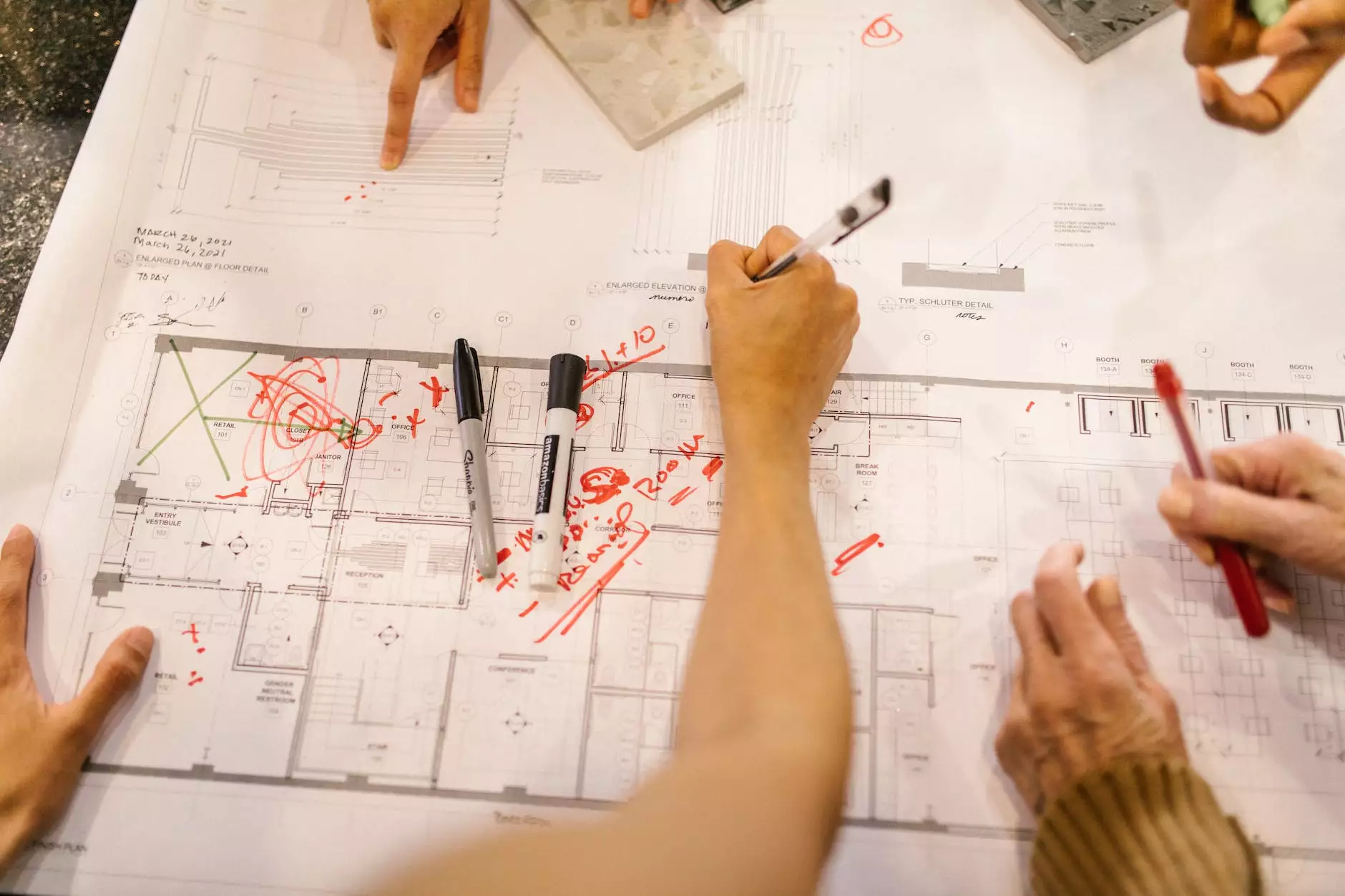
In the ever-evolving landscape of artificial intelligence (AI) and machine learning (ML), data annotation has become a pivotal process for transforming raw data into actionable insights. As businesses continue to harness the power of AI, they face the critical challenge of ensuring their datasets are accurately annotated. In this detailed guide, we'll explore the importance of data annotation, the tools and platforms available, and how solutions like https://keylabs.ai/ can serve your business needs.
What is Data Annotation?
Data annotation refers to the process of labeling data to make it understandable for machine learning algorithms. This involves the meticulous task of adding metadata or tags to various forms of data such as images, video, audio, and text. The quality and accuracy of data annotations play a crucial role in training machine learning models effectively.
Types of Data Annotation
- Image Annotation: This includes tasks like bounding boxes, segmentation, and landmark identification.
- Text Annotation: Text data can be annotated with sentiment tags, topic labels, or named entities.
- Audio Annotation: Involves labeling audio clips with transcriptions or speaker identification.
- Video Annotation: This is used for complex tasks like tracking objects through frames or classifying scenes.
The Importance of Data Annotation in AI and ML
Accurate data annotation is fundamental in developing high-quality machine learning models. Here’s why:
- Improved Model Accuracy: Data annotations guide ML algorithms in recognizing patterns, leading to better predictions and analyses.
- Enhanced Learning: Annotated datasets allow models to learn from examples, improving their ability to handle real-world scenarios.
- Streamlined Workflow: Efficient data annotation processes reduce time to market for AI applications, enhancing productivity.
- Compliance and Standards: Using precise annotations ensures compliance with data quality standards, critical for industries like healthcare and finance.
Challenges in Data Annotation
While data annotation is essential for successful AI projects, it comes with challenges, including:
- Expertise Requirements: Various types of data annotations require specialized knowledge, making it challenging to find qualified annotators.
- Scalability Issues: As projects grow, the volume of data to be annotated can become unmanageable for small teams.
- Quality Assurance: Maintaining consistency and accuracy in annotations can be difficult, especially over large datasets.
- Time-Consuming Processes: Manual annotation can be labor-intensive, with potential delays impacting project timelines.
Data Annotation Tools: An Overview
To overcome these challenges, a plethora of data annotation tools and platforms have emerged. These tools vary in functionality, scalability, and usability, catering to diverse needs. One of the notable platforms is https://keylabs.ai/, which offers comprehensive solutions designed to streamline the data annotation process.
Key Features of Data Annotation Tools
- Automated Annotation: Leveraging AI to partially or fully automate the annotation process, reducing time and labor costs.
- Collaboration Tools: Features that promote teamwork among annotators and project managers to enhance productivity.
- Multi-Format Support: Compatibility with various data formats, including image, video, text, and audio, adapting to diverse project needs.
- Quality Control Mechanisms: Built-in tools for verifying and validating annotations to ensure data quality.
- Scalability: Solutions that grow with your data needs, accommodating large datasets without compromising performance.
The Future of Data Annotation Platforms
As the demand for AI continues to rise, the future of data annotation platforms like https://keylabs.ai/ looks promising. Here are some anticipated trends:
1. Increased Automation
With advancements in machine learning, automation will play a dominant role in data annotation processes, making it faster and more efficient.
2. Crowdsourcing Solutions
Crowdsourced platforms may become more prevalent, leveraging the collective intelligence of a large number of contributors to annotate data effectively.
3. Enhanced User Interfaces
Technological advancements will lead to more intuitive and user-friendly interfaces, enabling annotators to perform tasks with greater ease and efficiency.
4. Real-Time Collaboration Tools
Future platforms will likely incorporate real-time collaboration features, allowing teams to work together seamlessly from different locations.
5. Integration with Other AI Tools
As businesses adopt AI for various functions, data annotation tools will increasingly integrate with other AI tools, providing a more holistic approach to data-driven projects.
Choosing the Right Data Annotation Tool for Your Business
When selecting a data annotation tool or platform, consider the following factors:
- Type of Data: Choose a platform that specializes in the type of data you need to annotate.
- Budget Constraints: Assess pricing models to find a solution that fits your budget without sacrificing quality.
- Scalability: Ensure that the tool can handle your current workload and future growth.
- User Reviews and Reputation: Research user experiences and testimonials to gauge the effectiveness of the tool.
- Support and Training: Opt for platforms that offer comprehensive support and training for smooth onboarding.
Conclusion
Data annotation is a cornerstone of successful AI and machine learning projects, transforming unstructured data into valuable insights. As we navigate the complexities of data annotation, platforms like https://keylabs.ai/ play a vital role in meeting the diverse needs of businesses. By understanding the intricacies of data annotation and leveraging the right tools, organizations can enhance their AI initiatives, improve outcomes, and ultimately achieve a competitive edge in their respective industries.
In the world of AI, where data is king, investing in quality data annotation solutions is not just an option; it’s a necessity for thriving in the digital age.