The Definitive Guide to Image Labeling
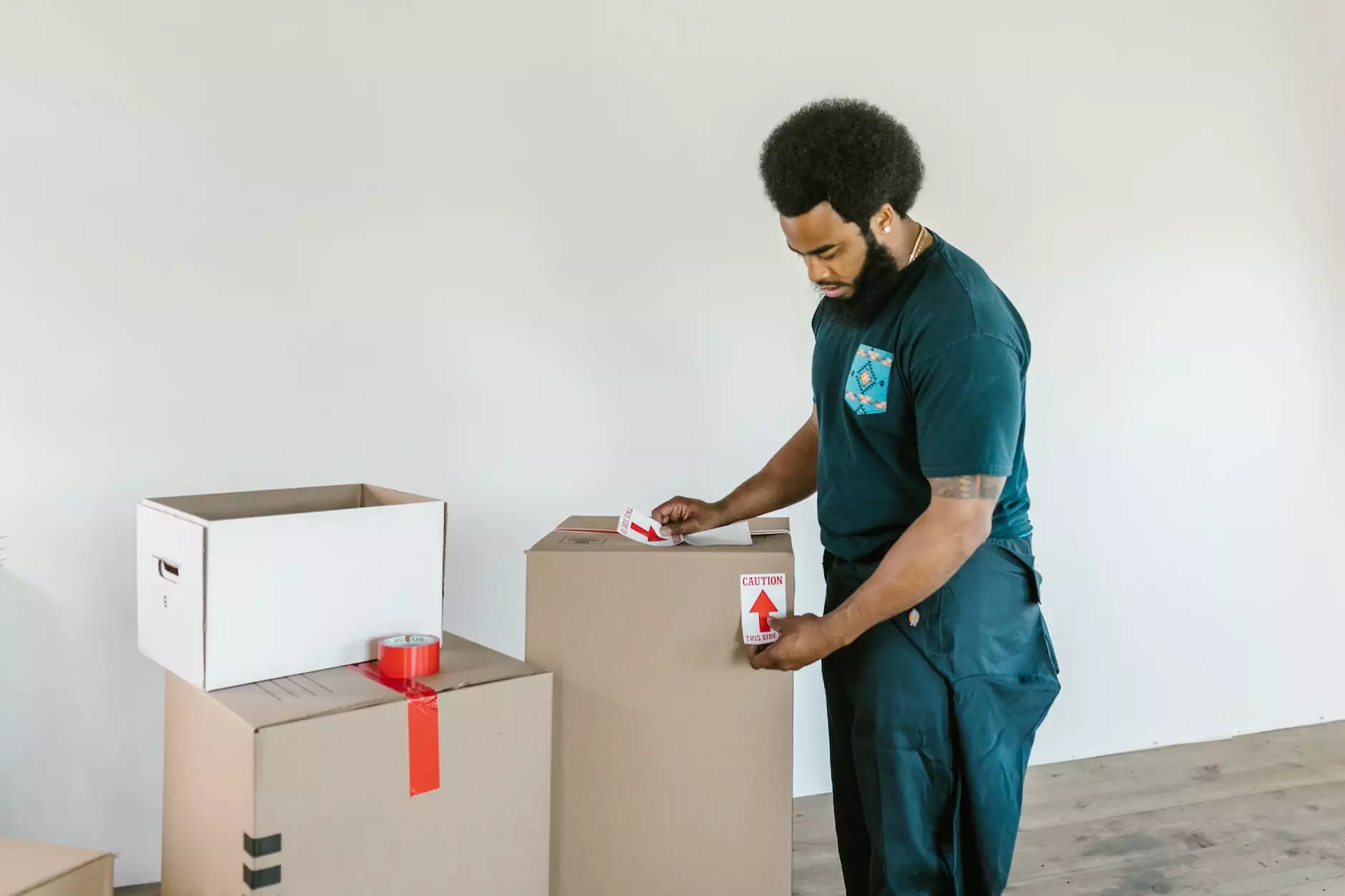
Image labeling is a crucial aspect of data annotation that has gained immense importance in the realm of artificial intelligence and machine learning. As businesses continue to leverage the power of AI, effective data preparation remains a foundational requirement for successful outcomes. This article will explore the various dimensions of image labeling, its significance in today's data-driven world, and how adopting the right practices can position your business at the forefront of technological innovation.
Understanding Image Labeling
Image labeling refers to the process of assigning tags or annotations to images, enabling machine learning models to understand the visual content effectively. The primary goal of image labeling is to facilitate the training of algorithms to recognize and classify objects within images accurately. This process involves detailing elements such as shapes, colors, and textual information, aiding in the development of intelligent systems capable of performing complex visual tasks.
Why Image Labeling Matters
In an era where data is the new oil, image labeling plays a vital role in optimizing the functionality of AI and enhancing business operations. Here are several key reasons why image labeling is indispensable:
- Enhances Accuracy: Proper labeling helps improve the precision of AI algorithms, leading to better predictions and classifications.
- Enables Automation: Well-labeled datasets enable automation in processes such as image recognition, freeing resources for more complex tasks.
- Facilitates Better User Experience: Accurate image labeling enhances applications like visual search engines and recommendation systems, improving the end-user experience.
- Supports Various Industries: From healthcare to autonomous vehicles, image labeling supports a multitude of sectors, driving innovation and efficiency.
The Process of Image Labeling
To implement effective image labeling, businesses must follow a structured approach. Here’s a detailed breakdown of the process:
1. Define Objectives
Establishing clear objectives is critical. Determine what you want to achieve with the labeled images—be it training a model for classification, detection, or segmentation.
2. Select the Right Tools
Choosing the appropriate data annotation tools can significantly impact the efficiency of the labeling process. At Keylabs.ai, we provide robust platforms designed to streamline your image labeling tasks.
3. Data Collection
Gather a comprehensive dataset of images relevant to your project. Ensure diversity in your dataset to account for various scenarios.
4. Begin Labeling
Utilize user-friendly annotation interfaces to start labeling your images. Depending on the complexity, you may opt for bounding boxes, segmentation masks, or keypoints to identify and outline objects.
5. Quality Assurance
Implementing quality control measures are paramount. Review labeled images to ensure consistency and accuracy, making corrections as needed.
6. Model Training
Once the labeling is complete, the data can be fed into machine learning models for training, ultimately allowing the system to learn and improve its recognition capabilities.
Types of Image Labeling Techniques
Understanding the different techniques for image labeling can empower businesses to choose the most suitable method for their needs. Here is an overview of common techniques:
1. Bounding Box Annotation
This technique involves drawing boxes around objects within images. It's widely used for tasks like object detection where the model needs to identify and locate objects within a scene.
2. Semantic Segmentation
Semantic segmentation divides an image into segments and labels each segment with a class. This method allows for pixel-level classification, which is beneficial for tasks requiring detailed object recognition.
3. Instance Segmentation
This approach goes a step further than semantic segmentation, distinguishing between different objects of the same class in a single image, which is vital for complex scenes.
4. Keypoint Annotation
Keypoint annotation focuses on marking specific points of interest in an image, commonly used in facial recognition technologies where critical features are identified.
Best Practices in Image Labeling
To achieve optimal results, it’s essential to follow best practices in image labeling:
- Consistency: Maintain consistency in labeling to avoid confusion for machine learning models.
- Clear Guidelines: Develop clear labeling guidelines for human annotators to ensure quality and uniformity in the labels.
- Utilize Automation: Leverage AI-assisted tools available at Keylabs.ai to reduce the manual workload and enhance productivity.
- Conduct Regular Audits: Regularly review labeled data to ensure accuracy and improve labeling quality.
- Incorporate Feedback: Engage users and stakeholders for feedback on the labeling process to continuously refine your practices.
The Role of Keylabs.ai in Image Labeling
Choosing the right partner for data annotation is crucial for your project's success. Keylabs.ai offers a comprehensive data annotation platform that simplifies image labeling while ensuring high quality and precision. Here’s what we bring to the table:
1. User-Friendly Interface
Our platform provides an intuitive interface that enhances the user experience, making the labeling process smooth and efficient for teams of any size.
2. Advanced Automation Tools
Keylabs.ai incorporates automation features that expedite the labeling process, allowing you to focus on model development rather than data preparation.
3. Scalability
Our solutions are designed to scale with your business needs, accommodating everything from small projects to enterprise-level datasets.
4. Quality Control
We implement strict quality control measures to ensure every labeled image meets your required standards, assisting in the creation of highly reliable models.
5. Dedicated Support
Our team of experts is always available to provide support and guidance, ensuring you have the resources you need to achieve your data labeling objectives.
Future Trends in Image Labeling
The field of image labeling is evolving rapidly, driven by technological advancements and increasing demand for sophisticated AI capabilities. Here are some emerging trends to watch:
1. Increased Use of AI in Annotation
AI-assisted automation tools are likely to become even more prevalent, allowing businesses to achieve greater efficiency in image labeling processes.
2. Focus on Quality Over Quantity
As the market matures, there will be a greater emphasis on creating high-quality labeled datasets, rather than simply amassing large volumes of data.
3. Integration with Other Technologies
We can expect more integration of image labeling tools with other tech stacks, such as cloud computing and big data analytics, creating more comprehensive solutions for data handling.
4. Ethical Considerations
As AI continues to permeate various aspects of society, there will be an increasing focus on ethics in image labeling, ensuring that models are trained responsibly and fairly.
Conclusion
In conclusion, image labeling is an integral part of the data annotation process that drives innovation across industries. By understanding its importance and implementing best practices, businesses can harness the power of AI more effectively. At Keylabs.ai, we are committed to providing cutting-edge solutions that empower organizations to navigate the complexities of data preparation seamlessly. As you embark on your data annotation journey, partnering with experts in image labeling will undoubtedly yield significant advantages, enhancing your competitive edge in the market.
With the knowledge gained from this article, you are now equipped to take decisive steps towards optimizing your data annotation practices, ensuring your business thrives in a data-centric landscape.